The Rise of Artificial Intelligence in Healthcare: Why Critical Thinking Matters
Artificial Intelligence (AI) is rapidly transforming healthcare, offering powerful tools that not only streamline diagnostics, but also hold the potential to revolutionize the healthcare industry. From predictive analytics to automated clinical decision support, AI systems promise to augment human expertise and optimize patient care. However, a growing challenge that comes with these advancements is making sure that critical thinking stays at the center of medical decision-making.
As AI systems become more autonomous, there is a risk that clinicians will rely too heavily on algorithmic recommendations, a phenomenon known as automation bias. Research suggests that Decision Support Systems (DSS) can unintentionally encourage cognitive offloading where users defer too much judgment to AI, reducing their independent analytical engagement. This poses a crucial query: How can medical practitioners incorporate AI into their procedures without compromising their judgment and oversight?
This article explores that balance. We believe that while AI can serve as a valuable tool, it must not replace the clinician’s ability to assess, question, and interpret its recommendations. Studies indicate that AI explanations often fail to align with physician expectations, making it even more crucial for healthcare professionals to refine and maintain their critical thinking skills. The goal here is not to reject AI but to use it wisely—leveraging its strengths while maintaining accountability and independent judgment.
Understanding AI’s Role in Healthcare: Efficiency Gains and Emerging Risks
Artificial Intelligence is reshaping healthcare workflows, offering unprecedented clinical and administrative task efficiency. From automating documentation to synthesizing vast medical literature, AI-powered tools provide healthcare professionals with rapid access to critical information. In nursing, for example, AI models have demonstrated their ability to assist in triage by structuring clinical decision-making and prioritizing patient needs. Similarly, radiologists benefit from AI-driven image analysis, where algorithms quickly highlight potential areas of concern, expediting the diagnostic process. These innovations enhance productivity and allow clinicians to focus more on direct patient care.
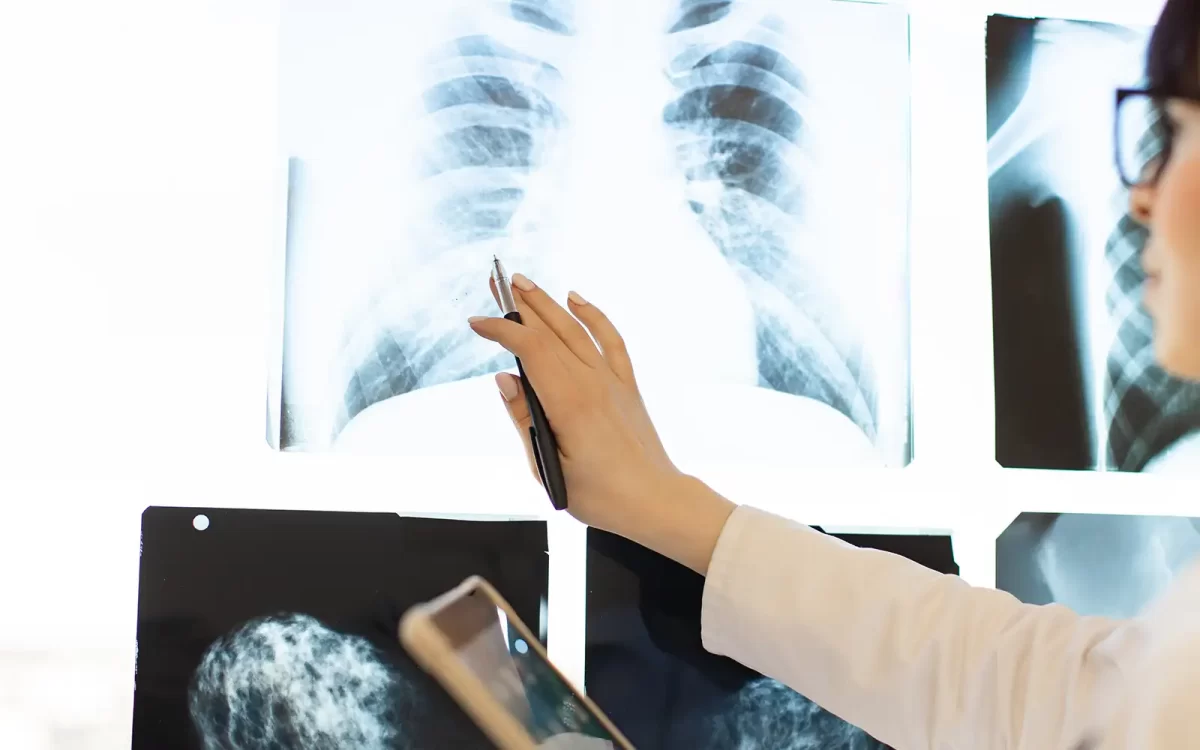
Nevertheless, there are risks associated with these efficiency gains. One of the most pressing concerns is over-reliance on AI, where clinicians may accept AI-generated recommendations without sufficient scrutiny. This can lead to a cognitive shortcut that weakens independent evaluation skills over time. The phenomenon is compounded by AI’s occasional tendency to generate incorrect or misleading outputs, often referred to as hallucinations. For instance, studies have found that AI-assisted triage models sometimes recommend unnecessary tests, leading to inefficiencies in resource allocation.
Beyond factual inaccuracies, AI’s opaque decision-making process is also challenging. Many machine learning models function as “black boxes” meaning their reasoning is not always interpretable to human users. When clinicians cannot fully assess why an AI system arrives at a particular conclusion, there is a risk of uncritical acceptance—trusting the output without questioning its validity. In an environment where medical errors can have life-altering consequences, the ability to critically evaluate AI-generated insights is essential.
As AI continues to integrate into healthcare, professionals must remain vigilant, ensuring that these tools serve as aids rather than replacements for human expertise. Maintaining a balance between efficiency and autonomous clinical reasoning will require not only utilizing AI’s advantages but also acknowledging its limitations.
The Impact of AI on Critical Thinking: A Double-Edged Sword
AI’s growing role in healthcare is reshaping not only workflows but also how clinicians approach decision-making. Studies suggest increased trust in AI can lead to a subtle yet significant shift in cognitive effort from active problem-solving to verification and integration. While AI’s ability to rapidly synthesize information reduces the burden of recall and comprehension, this convenience comes with the cost of diminished critical thinking.
Research shows that clinicians receiving AI-generated recommendations are more likely to accept them without deep scrutiny, particularly in high-pressure environments. Novice nurses, for example, have been found to defer more readily to AI-assisted triage suggestions, prioritizing verification over independent reasoning. This reflects a broader trend: as AI delivers fast, structured answers, professionals may unconsciously engage less in the analytical reasoning that defines expert judgment. The issue at hand is not simply whether AI will make mistakes; rather, it is whether or not clinicians will continue to actively identify them and use AI as a tool rather than a decision-maker.
This shift in cognitive effort has real consequences in daily practice. In surgical settings, for instance, reliance on AI-assisted robotics raises concerns about the erosion of hands-on skills. If surgeons defer too much to automation, their ability to operate without AI support may weaken. The same risk extends to diagnostic reasoning and clinical assessments, where habitual dependence on AI-generated healthcare insights could gradually diminish practitioners’ critical thinking ability in unexpected or ambiguous cases.
What is the key takeaway? AI should complement human expertise, not replace it. The true difficulty is finding a balance between utilizing AI’s effectiveness and maintaining the critical, introspective thinking that characterizes clinical excellence. The future of healthcare will not be about picking between artificial intelligence and human judgment, but rather about making sure that each improves the other. This balance is crucial to ensure that AI is used as a support tool, not a decision-maker.
Self-Assessment: Knowing Your Baseline
The first step in strengthening critical thinking alongside AI is understanding how much we already rely on it—and where that reliance may be creeping in unnoticed. In fast-paced clinical settings, AI tools often serve as an immediate source of structured information, streamlining documentation, diagnostics, and decision-making.
Under time pressure, clinicians are more likely to accept AI recommendations without deeper investigation, favoring efficiency over caution. This tendency can be subtle—skipping a manual verification step, assuming an AI-generated summary is complete, or adjusting clinical decisions based on AI predictions without second-guessing them. In this case, self-reflection is crucial: Are we using AI as a tool for support, or are we letting it dictate our decisions?
One effective strategy is to adopt reflection prompts—structured questions designed to challenge AI outputs before accepting them. This approach forces a moment of pause, encouraging deeper engagement with the information rather than passive acceptance. This habit-building, in our opinion, is essential; the objective is to promote an attitude of active inquiry rather than merely identify AI errors.
Strategies for Enhancing Personal Mastery in AI-assisted Decision making
AI is a powerful tool, but independent clinical reasoning requires intentional strategies. Strengthening verification habits, reducing overreliance on AI, and continuously refining decision-making skills can ensure that AI enhances rather than substitutes for expertise.
Improving Verification and Integration Skills
A key defense against automation bias in healthcare is developing a habit of cross-checking AI-generated recommendations. Research suggests that structured reflection—actively questioning AI outputs—can reinforce critical thinking. One effective approach is adopting a two-source verification process: before acting on AI suggestions, compare them with trusted clinical guidelines, peer-reviewed literature, or personal expertise. This method shifts AI from an unquestioned authority to a starting point for deeper analysis.
Another strategy is using explainable AI (XAI) tools when available. Systems designed with transparency allow clinicians to trace AI reasoning, making it easier to assess whether an output aligns with medical best practices. Even when AI recommendations appear sound, engaging in systematic validation—asking, Does this align with my clinical understanding? What evidence supports this decision?—can prevent passive acceptance.
Reducing Over-Reliance on AI
Blind trust in AI erodes autonomy. One way to counteract this is by forming an independent clinical judgment before consulting AI recommendations. Studies show that clinicians making an initial decision before seeing AI-generated advice are more likely to critically assess discrepancies rather than default to AI conclusions. This practice reinforces self-reliance and ensures that AI is not a full decision-maker.
Additionally, maintaining the ability and confidence to override AI is essential. Some AI models present recommendations with an authoritative tone, making it psychologically difficult to disagree. Clinicians should actively remind themselves that AI, like any tool, has limitations. Committing to periodic AI-free decision-making exercises—handling cases without AI assistance—can help preserve the sharpness of independent reasoning.
Building Continuous Learning
AI is not static, and neither should our understanding of it. One way to improve both AI literacy and clinical reasoning is through AI-driven simulations. Case-based learning, where AI generates clinical scenarios for practitioners to assess and critique, offers a safe space to refine diagnostic and decision-making skills. Studies highlight that such simulations allow professionals to engage with AI critically, using it as an interactive learning partner rather than an infallible source.
Further, clinicians should seek ongoing education on AI’s evolving capabilities, biases, and limitations. Reviewing post hoc explanations—why an AI system made a particular recommendation—can provide insights into its decision-making patterns. Over time, this engagement fosters a deeper understanding of AI’s strengths and weaknesses.
Practical Tools and Techniques for Daily Practice of AI Healthcare Solutions
Creating and Using Checklists
Checklists serve as cognitive guardrails, helping clinicians verify AI outputs with structured questioning. Research suggests that structured counterarguments can prompt clinicians to systematically question AI-generated recommendations.
A well-designed verification checklist might include:
-
Does the AI recommendation align with the patient’s entire medical history?
-
Does it match established clinical guidelines?
-
Have alternative explanations been considered?
-
Would the same decision be made without AI input?
Implementing Cognitive Timeouts
Fast-paced clinical environments often encourage snap decisions, especially when AI provides immediate answers. However, we must highlight the importance of cognitive timeouts—deliberate pauses to reflect before finalizing a decision. This technique is particularly relevant in high-risk scenarios, such as AI-assisted robotic surgery, where surgeons are encouraged to confirm each AI-driven step before proceeding.
A practical way to implement this is by embedding sequential decision-making into workflows. Before accepting an AI recommendation, clinicians can allocate a specific moment to re-evaluate key factors:
-
What assumptions is the AI making?
-
Are there any gaps in the AI’s reasoning?
-
What would be done if the AI were unavailable?
This short but intentional pause can help counter automation bias in healthcare, ensuring clinicians remain active participants in decision-making.
Engaging in Peer Collaboration
Multi-factor verification—having multiple clinicians review AI-generated recommendations—can help catch biases and prevent cognitive shortcuts. Encouraging second opinions, whether informally through peer discussions or structured review processes, fosters a culture of collective oversight.
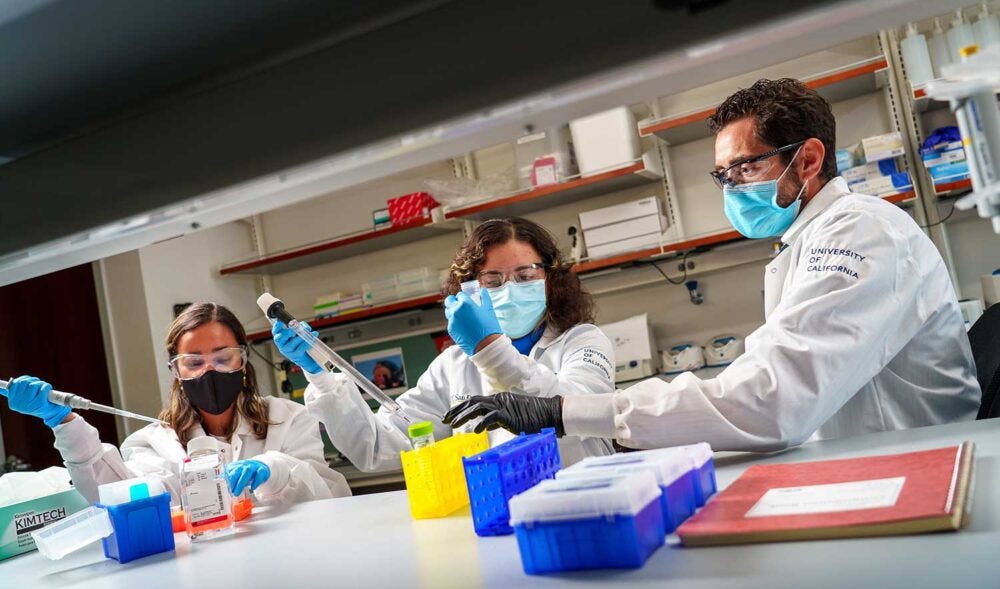
For instance, in complex diagnostic cases, a “second opinion” workflow could involve:
- The primary clinician forms an independent assessment.
- AI generates its own recommendations.
- A peer review is required before a final decision is made.
Such collaborative reviews help counteract individual biases and reinforce human oversight in AI-assisted decision-making.
Leveraging Integrated Resources
To avoid blind reliance on AI, clinicians should have immediate access to trusted medical resources for verification. AI systems that integrate retrieval-augmented fact-checking—linking outputs to databases like UpToDate, PubMed, or clinical guidelines—can help bridge the gap between automation and human expertise.
For example, explainable AI (XAI) models embedded in electronic health records (EHRs) allow clinicians to cross-reference AI suggestions with evidence-based guidelines. Rather than taking AI outputs at face value, clinicians can quickly assess how recommendations align with established medical knowledge.
Balancing Efficiency and Critical Thinking
Optimizing Workflows
Used correctly, AI can reduce administrative burdens, allowing clinicians to focus more on complex decision-making. In triage, for example, AI models have been shown to accelerate task prioritization, enabling nurses to devote more time to patient care efficiency. Similarly, radiologists benefit from AI’s ability to highlight areas of concern in imaging, improving turnaround times. These speed increases must be carefully controlled, though, as there is a greater chance of overlooking important details when efficiency breeds over-reliance.
A strategic approach is to let AI handle preliminary assessments while clinicians perform the final evaluations. For example:
- AI can generate structured clinical notes, but practitioners should always review and refine them.
- AI can flag abnormalities in imaging, but radiologists must ensure they examine all relevant areas, not just AI-identified regions.
- AI can offer differential diagnoses, but clinicians should independently weigh patient history, symptoms, and test results before deciding.
By using AI as a workflow optimizer rather than a decision-maker, clinicians can improve efficiency while actively engaging in medical reasoning.
Maintaining Hands-On Skills
One of AI’s hidden risks is the gradual erosion of manual expertise. In surgical fields, for instance, overreliance on robotic assistance can lead to a loss of critical procedural skills. Similarly, AI-generated assessments can diminish the instinctive pattern recognition from experience in diagnostic medicine. Research warns that without deliberate practice, core clinical skills can weaken over time.
To counteract this, clinicians should regularly engage in:
-
Case Reviews – Analyzing cases independently before consulting AI-generated insights.
-
Hands-On Practice – Performing manual diagnostics, procedural tasks, and physical examinations without AI assistance.
-
Diagnostic Discussions – Engaging in peer-based case studies to reinforce reasoning without algorithmic input.List Item
AI should serve as a second opinion, not the first instinct. Ensuring clinical judgment remains sharp requires regular engagement with cases beyond AI-driven suggestions.
Time Management Strategies
One of AI’s paradoxes is that while it saves time, it can also introduce pressure to move too quickly. Studies from other fields suggest that real-time AI tools can create a false sense of urgency, blurring the boundaries between speed and accuracy. The same applies in healthcare—if AI-generated recommendations are always immediately available, there may be less inclination to step back and reassess decisions thoroughly.
To prevent this, clinicians can:
-
Schedule Dedicated “Offline” Review Time – Setting aside moments in the day for deeper, independent evaluation of AI-generated insights.
-
Use Cognitive Timeouts – Implementing structured pauses before deciding to reflect on alternative explanations.
-
Prioritize Complex Cases for Human-Driven Analysis: Reserve manual review for high-risk or ambiguous cases where AI errors could be particularly costly.
AI should create more room for thoughtful evaluation and not push clinicians toward faster, less reflective decisions. Structuring time intentionally ensures that efficiency gains do not undermine careful clinical reasoning.
Ethical and Professional Considerations
Understanding Responsibility
The integration of AI in healthcare does not shift liability away from clinicians. Even when AI contributes to decision-making, physicians remain the final authority responsible for patient outcomes. In surgical settings, for instance, AI-assisted robotic systems can guide precision movements, but if an error occurs, accountability can still fall on the surgeon. This legal and ethical reality highlights the need for informed engagement with AI rather than passive reliance.
Beyond personal responsibility, clinicians must also consider AI’s implications for patient autonomy. As AI-driven recommendations become more common, the principle of informed consent must evolve to ensure that patients understand when and how AI is involved in their care. It is imperative to be transparent about AI’s limitations as well as its potential. Patients should know that AI provides recommendations, but human oversight remains the final safeguard.
Learning from Real-World Examples
Case Studies and Lessons Learned
One case involved an AI model designed to assist in managing chronic low back pain (CLBP). Initially, the system recommended conservative treatment based on general patterns in patient data. However, a Reflection Machine prompt encouraged clinicians to reevaluate the AI’s suggestion. Upon closer review, they identified a structural spinal issue that warranted surgical intervention, something the AI had overlooked. This example highlights the importance of structured questioning in preventing diagnostic tunnel vision and ensuring that AI does not narrow clinical possibilities prematurely.
In hindsight, cases such as this could have been managed more efficiently if clinicians had applied a verification step—comparing AI-driven suggestions against established protocols before proceeding. This reinforces the need for a pause-and-check habit, particularly in time-sensitive environments where defaulting to AI can be tempting. Not all AI-related errors are dramatic, but even small misjudgments can have significant consequences. Consider AI-driven medication deprescribing, studies show that AI can flag patients for deprescription based on broad clinical criteria. Still, without human review, it may miss key contextual factors—such as withdrawal risks or patient-specific contraindications. When physicians reflect on these cases, they often recognize moments where independent judgment could have refined or overridden AI’s initial suggestions.
Personal Reflection
One of the most effective ways to improve AI-integrated decision-making is through self-assessment. Reviewing past successful and problematic cases can help identify patterns in how AI influences clinical reasoning.
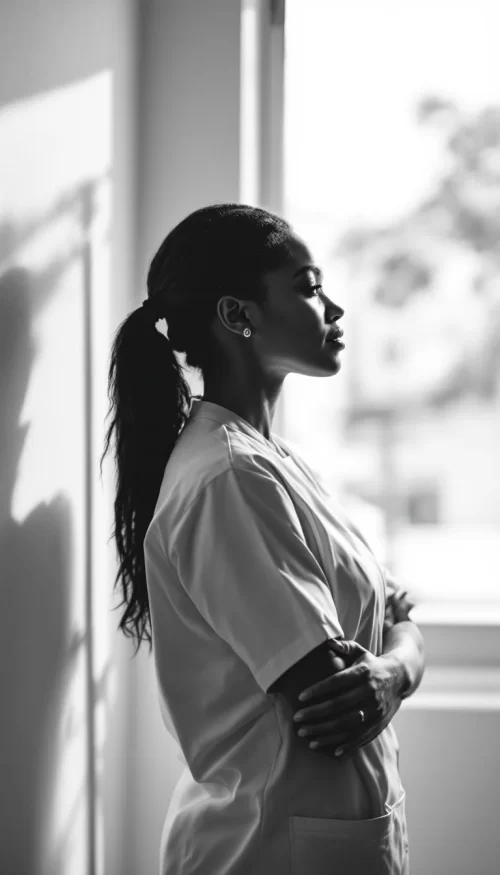
Questions to consider include:
-
When did I accept an AI recommendation without deeper scrutiny?
-
Were there instances where AI’s suggestion contradicted my initial instincts? How did I respond?
-
Have I noticed a pattern when I am most likely to trust AI (e.g., under time pressure, in unfamiliar cases)?
Reflecting on these questions can uncover cognitive biases shaping how to balance AI and human expertise in healthcare. Research suggests that awareness of these biases is the first step toward mitigating them.
Action Plan for Personal Development
Integrating AI into clinical decision-making requires an intentional approach to maintaining critical thinking skills. By setting clear goals, implementing structured verification processes, and continuously monitoring progress, healthcare professionals can ensure that AI enhances their expertise without diminishing independent judgment.
Setting Clear Goals
To use AI effectively, clinicians should establish concrete objectives for improving AI literacy and critical evaluation skills.
Some key goals might include:
-
Developing AI Literacy: Learn how AI models generate recommendations, their limitations, and their biases.
-
Understanding AI Confidence Scores: Identify when AI is uncertain and how to weigh its recommendations accordingly.
-
Refining Verification Skills: Implement a consistent method for cross-checking AI suggestions with clinical guidelines and peer-reviewed evidence.
By setting specific, measurable goals, clinicians can track their growth in navigating AI-assisted decision-making with confidence and precision.
Step-by-Step Implementation
Building stronger AI-related critical thinking habits requires a structured approach.
A stepwise plan might include:
- Integrating Reflection Prompts into Daily Practice
- Before accepting an AI recommendation, ask: Does this align with clinical best practices? What alternative explanations exist?
- Use Reflection Machines or cognitive timeouts to systematically challenge AI-generated insights.
- Establishing a Verification Workflow
- Adopt a two-source validation process: compare AI-generated insights with trusted medical guidelines, peer-reviewed research, or a second opinion.
- For high-impact decisions, implement human audits—ensuring a manual review before acting on AI recommendations.
- Scheduling Regular Self-Assessment and Peer Review Sessions
- Set aside time to review past cases where AI was used—evaluating whether its recommendations were accurate and whether personal judgment was applied effectively.
- Engage in peer discussions to refine critical evaluation skills and expose potential cognitive biases.
Monitoring Progress
Maintaining strong critical thinking skills in an AI-driven environment requires ongoing assessment. Without intentional monitoring, there is a risk of over-reliance creep, which is the gradual reliance on AI without realizing that independent judgment is waning.
Strategies for tracking progress include:
-
Feedback Loops: Regular discussions with supervisors and colleagues can reveal whether AI enhances or undermines decision-making.
-
Verification Tracking: Monitor how often AI-generated suggestions are verified or overridden—identifying trends that may indicate a shift toward passive acceptance.
-
Case-Based Reflection: Periodically review past clinical decisions to evaluate whether AI was used appropriately or whether independent reasoning could have been stronger.
Final Thoughts on How to Improve Independent Reasoning in an AI-driven world
The integration of AI in healthcare presents both opportunities and challenges. AI can streamline workflows, enhance efficiency, and provide valuable insights, but it must never replace the clinician’s independent judgment. As research confirms, AI is most effective when paired with active and effective human oversight, where verification and critical thinking remain central to decision-making. Without these safeguards, there remains a risk of automation bias.
Maintaining autonomy in clinical practice requires continuous effort. Reflection prompts, cognitive timeouts, and structured verification processes ensure that AI remains a tool for enhancement rather than a substitute for expertise. Furthermore, regular self-assessment and peer collaboration foster a culture of accountability in which AI recommendations are challenged rather than blindly accepted.
In the end, rather than acting as an authority, AI should be a collaborator in clinical care. We believe that the future of medicine will not be defined by AI’s capabilities alone but by how well clinicians engage with AI critically and responsibly. The key is to remain vigilant—actively questioning, verifying, and refining AI’s contributions—to ensure that human expertise remains at the heart of patient care.