Ensuring AI Enhances—Not Replaces—Clinical Judgment
The expanding role of Artificial Intelligence (AI) in healthcare technology impacts clinical decision-making. AI models can now assist with diagnoses, treatment planning, and administrative efficiency, accelerating workflows that once demanded hours of manual effort. As one leans toward these developments, a crucial query arises: How do we ensure that clinicians remain active evaluators rather than passive recipients of AI-generated recommendations?
Research shows that human oversight diminishes when AI systems provide direct answers without encouraging scrutiny. Clinicians may lean on AI outputs without questioning their validity, eroding accountability in high-stakes medical decisions. Case studies illustrate that excessive reliance on AI-driven clinical decision support can lead to overlooked errors when recommendations go unchallenged in fields like radiology and diagnostics. If designed poorly, AI can create a false sense of certainty.
This article is a guide for AI developers. The objective isn’t merely to build powerful algorithms but to design systems that integrate seamlessly into clinical workflows while reinforcing critical thinking. AI should support decision-making, not replace it. The key lies in structuring AI interactions that require verification, encouraging clinicians to engage with recommendations rather than accept them at face value. The challenge is striking the right balance—leveraging AI’s efficiency while ensuring that human expertise remains the final authority in patient care.
This is the second article of our series on supporting critical thinking in healthcare decision making in a world dominated by Automation and AI. The first piece looked at what organizations can do to support critical thinking in this environment. You can read it here.
The Role of AI in Health care
AI has already begun reshaping healthcare, offering substantial benefits in efficiency, knowledge retrieval, and diagnostic support. Yet, alongside these advantages, the risks of overreliance and erroneous outputs pose serious challenges. Striking a balance between automation and human oversight is critical, as AI should serve as an aid, not an unquestioned authority.
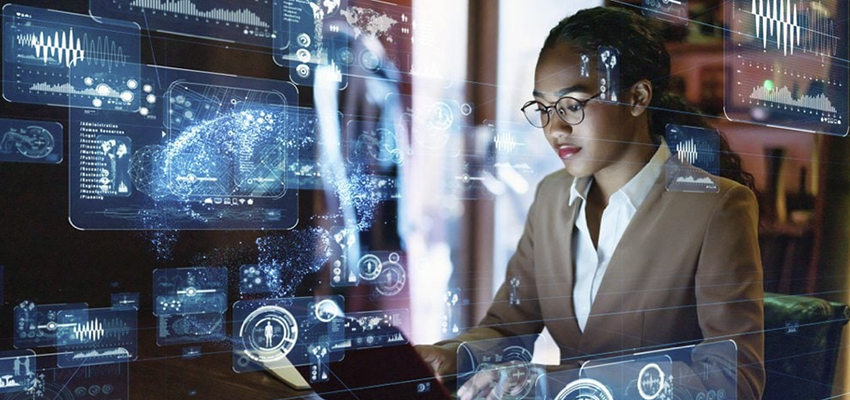
Inherent Risks: The Dangers of Overreliance
A primary concern is automation bias in AI; this is the tendency to trust AI recommendations when we shouldn’t. Research indicates that when clinicians repeatedly interact with AI-generated suggestions, they may begin to offload cognitive responsibility, gradually reducing their own independent reasoning. This can be particularly problematic in high-stakes decisions, where AI outputs, if unverified, could lead to inappropriate clinical actions.
Another challenge is AI hallucination in healthcare, which occurs when AI generates plausible-sounding but incorrect or misleading information. These errors can be subtle, especially in nuanced areas like medication adjustments or complex case management. Case studies suggest that when even AI provides vague or inaccurate recommendations, clinicians may unconsciously conform to them rather than question their validity, increasing the risk of suboptimal patient care.
While AI has the potential to significantly enhance healthcare, its design must ensure that clinicians remain engaged and critical thinkers. The next step is understanding how AI systems can be structured to reinforce and not replace clinical judgment.
Key Study Findings on Critical Thinking and AI
While AI offers efficiency gains, research suggests a crucial tradeoff: independent critical thinking may decline as confidence in AI increases. Instead of actively solving problems, clinicians may shift toward verifying AI-generated recommendations—a subtle but profound transformation in cognitive behavior.
Impact on Critical Thinking: A Shift from Problem-Solving to Verification
Studies indicate that users become less likely to engage in deeper analysis when AI tools are perceived as highly reliable. Instead of working through complex cases from first principles, clinicians may focus on confirming AI-generated healthcare insights. This shift isn’t inherently negative, as verification is a valuable cognitive task but carries risks.
Efficiency-Accountability Trade-off
This efficiency-accountability tradeoff is particularly pronounced in time-sensitive environments. When AI streamlines decision-making, the temptation to trust its outputs without full examination grows. AI reduces cognitive workload, it also alters how clinicians allocate mental effort, sometimes reinforcing speed over depth. The result is a subtle erosion of independent judgment, where AI serves as an implicit decision-maker.
Specific Challenges in Healthcare: The Risks of Over-Reliance on AI-driven clinical Decision support
Overreliance on AI can be particularly dangerous in high-stakes fields like surgery and critical care. When AI-driven recommendations are accepted without question, subtle errors—such as incorrect dosage calculations or misinterpretations of imaging data—can have serious consequences. Even in advanced AI systems designed to mitigate automation bias in AI, human verification remains essential to catch mistakes that might go unnoticed.
This underscores the fundamental reality that AI is not infallible. While it can enhance clinical workflows, its outputs must always be subject to human oversight. The main task facing AI developers today is to create systems that support critical thinking rather than take its place, making sure that verification is still an intentional and active procedure rather than a passive formality.
The Developer’s Responsibility in AI Design
AI in healthcare should serve as an assistant, not an authority. While it can enhance clinical workflows, it must support and refine human judgment rather than dictate decisions. This responsibility falls heavily on developers, who must design systems encouraging active evaluation rather than passive acceptance. Promoting autonomy, preventing AI bias in medicine, improving AI transparency in medical decision-making and explainability are crucial steps in making sure AI serves as a guide rather than a final arbiter.
Ensuring AI as an Assistant, Not an Authority
Clinicians should feel empowered by AI, not bound by it. Systems that present a single “correct” answer risk undermining physician autonomy, making it too easy for users to defer without scrutiny. Instead, AI should offer multiple recommendations, each with supporting context, allowing clinicians to compare options rather than simply confirm an AI-generated conclusion.
One practical approach is designing AI interfaces that prompt clinicians to form an initial opinion before viewing AI outputs. This strategy disrupts reflexive agreement, ensuring that AI serves as a second opinion rather than the primary source of decision-making. Additionally, rather than presenting recommendations outright, AI systems can first provide structured guidance—such as highlighting key considerations—before revealing specific suggestions. These design choices reinforce human agency, positioning AI as a decision-support tool rather than a decision-maker.
Promoting Transparency and Explainability
For AI to be trusted, it must be understandable. Explainable AI in healthcare (XAI) enhances adoption by making its reasoning visible, allowing clinicians to evaluate not just the output but the logic behind it. Developers can achieve this by incorporating features that clarify how AI arrived at a given recommendation, such as identifying the data sources or breaking down the decision-making process into intuitive steps.
When AI-generated healthcare insights are paired with clear explanations, clinicians are more likely to engage critically with the information rather than simply accepting it at face value. This transparency also safeguards against biased or misleading outputs—if users can trace the reasoning behind AI suggestions, they are better equipped to challenge inconsistencies or errors.
Developers can create systems that enhance decision-making without diminishing human expertise by designing AI that prioritizes clinician involvement and interpretability. The challenge is implementing these principles in real-world AI applications, ensuring that every AI-assisted decision remains a collaboration, not a substitution.
Design Strategies to Support Clinical Judgment
AI systems must do more than generate recommendations—they should reinforce clinicians’ ability to think critically, verify outputs, and integrate AI suggestions into a broader clinical context. Effective AI design encourages users to interact with the logic behind the answers rather than merely offering them. This requires intentional design strategies that enhance verification, minimize over-reliance, and ensure transparency in AI-assisted decision-making.
Enhancing Verification and Integration
AI should function as an extension of evidence-based medicine, not a black box that clinicians must either trust or reject. One way to reinforce verification is by embedding real-time access to trusted medical sources such as UpToDate, PubMed, or clinical guidelines directly within AI tools. When AI-generated recommendations are accompanied by citations or supporting literature, clinicians can cross-check information without disrupting workflow.
Equally important is the design of AI interfaces. Instead of displaying recommendations as definitive answers, interfaces should encourage active evaluation by prompting clinicians to compare AI-generated insights with patient-specific data. Simple design choices, like side-by-side displays of AI suggestions and source references, can subtly nudge users toward verification rather than blind acceptance.
Minimizing Over-Reliance on AI in Healthcare
The implementation of “cognitive timeout” features, which require clinicians to pause and review AI recommendations prior to making critical decisions, can help clinicians avoid automation bias. This is especially helpful in areas where instant trust in AI outputs could have serious repercussions, such as emergency medicine or surgery.
Additionally, AI systems can be designed to require explicit user confirmation before proceeding. This could be a structured review process where clinicians must check key aspects of AI-generated suggestions such as alignment with patient history, clinical relevance, or potential risks—before acting on them. These friction points ensure that AI remains a tool for augmentation, not unquestioned automation.
Providing Multiple Alternatives
One of the most effective ways to prevent over-reliance is to avoid presenting AI outputs as singular truths. Instead, AI should generate multiple potential recommendations, each accompanied by pros, cons, and levels of certainty. For instance, AI could propose three differential diagnoses in diagnostic support, explaining the reasoning and data confidence behind each. By doing so, the system encourages clinicians to weigh different possibilities rather than defer to a single AI-generated conclusion.
Furthermore, AI should be explicit about uncertainties or limitations in its suggestions. Suppose an AI model is less confident in a particular case due to limited data. This uncertainty should be made transparent in that case, prompting clinicians to apply their expertise rather than assume completeness.
User-Friendly Verification Tools
AI systems should provide built-in checklists and structured prompts that guide clinicians through critically evaluating AI outputs. These prompts could include key verification questions such as:
✔ Does this recommendation align with the patient’s entire clinical history?
✔ Have alternative explanations been considered?
✔ What level of confidence does the AI assign to this suggestion?
Beyond checklists, on-demand access to explanations and data sources is essential. A well-designed AI interface should allow users to drill down into the rationale behind a recommendation, view supporting literature, and even adjust parameters to see how different inputs affect the output.
AI developers can create systems that strengthen rather than undermine clinical judgment by focusing on verification, thoughtful interaction, and transparency. The objective is to keep clinicians involved and critical thinkers in the decision-making process, not just to increase AI accuracy.
Addressing Developer Challenges and Ethical Considerations
Building AI systems for healthcare is not just a technical challenge—it’s an ethical and regulatory responsibility. Developers must navigate issues of bias, transparency, and accountability, ensuring that AI enhances clinical decision-making without compromising safety or trust. This requires continuous refinement of models, active engagement with users, and clear delineation of legal responsibilities.
Bias and Data Quality: Ensuring Fairness and Accuracy
AI is only as reliable as the data it learns from, and biased training data can lead to dangerously skewed recommendations. AI models trained on incomplete or non-representative datasets can produce erroneous suggestions, disproportionately affecting underrepresented patient groups. To mitigate this, developers must proactively audit and refine datasets, ensuring diverse demographic coverage and regularly testing AI outputs for bias-related disparities.
Beyond initial training, systematic updates are essential. Bias isn’t a static issue—it evolves as new data becomes available. AI tools must undergo continuous bias checks and recalibrations throughout their lifecycle, with mechanisms in place to flag and correct deviations in performance. Transparency in dataset composition and clear documentation of AI decision-making pathways can further strengthen trust among clinicians.
User Feedback and Iterative Improvement: Closing the Loop
AI models should not be static entities but evolve based on real-world clinical experience. One of the most effective ways to refine AI performance is through direct user feedback loops. When clinicians provide feedback on AI-generated suggestions—especially ambiguous or irrelevant recommendations, developers can fine-tune algorithms to reduce false positives and improve contextual accuracy.
Practical implementations include in-app reporting tools that allow clinicians to flag questionable AI outputs, suggest modifications, or rate the usefulness of recommendations. This feedback can be incorporated into algorithm threshold adjustments, helping AI systems become more attuned to real-world medical complexities. Additionally, regular developer-clinician collaborations, such as iterative testing phases, can ensure that AI tools align with actual clinical workflows rather than theoretical optimizations.
Legal and Ethical Responsibilities: Clarifying Accountability
AI in healthcare cannot function as an autonomous decision-maker—ultimate responsibility must always remain with clinicians. The boundaries of accountability, however, may blur as AI tools are used more frequently. Developers must ensure that AI-generated recommendations are framed as advisory, not authoritative, reinforcing that human oversight is an essential safeguard.
Clear guidelines and disclaimers should be embedded in AI interfaces, explicitly stating that recommendations are decision-support tools, not definitive directives. Regulatory expectations are also evolving, requiring greater transparency in AI models—from how data is processed to how final outputs are derived. In order to meet these new standards, developers must focus on designing ethical AI for healthcare that integrates explainability, oversight procedures, and compliance frameworks from the beginning.
Collaborative Development with Healthcare Stakeholders
To be truly effective in clinical settings, AI must be built in close collaboration with the professionals who use it. AI developers cannot design in isolation—real-world clinical workflows, constraints, and decision-making processes must shape how these tools function. Developers can ensure that AI systems are practical and trusted by engaging clinicians early in the design process and fostering AI literacy.
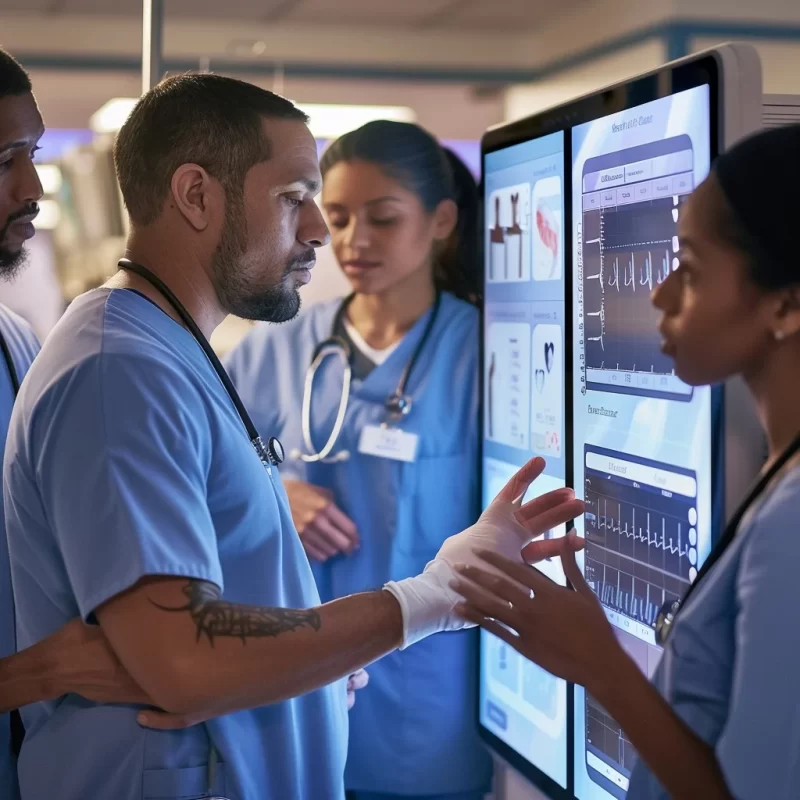
Engaging Clinicians in the Design Process AI in Healthcare
The best AI tools are not imposed on healthcare professionals—they are co-created with them. This means going beyond theoretical optimizations and engaging directly with those who will use AI in high-stakes environments.
Developers should establish regular focus groups, beta-testing sessions, and iterative design reviews with physicians, nurses, and specialists. These collaborative sessions can uncover pain points in existing workflows, allowing AI to be designed as a seamless enhancement rather than a disruptive addition. Studies also highlight the value of brainstorming sessions, where clinicians provide feedback on AI-generated prompts, ensuring that recommendations encourage reflection rather than passive acceptance.
A particularly effective approach is embedding developers within clinical environments and observing how decisions are made in real time. This direct exposure helps developers grasp the contextual complexity of medical decision-making, from subtle diagnostic reasoning to emergency care pressures. When clinicians and developers work side by side, AI tools become smarter, more intuitive, and truly supportive of clinical expertise.A particularly effective approach is embedding developers within clinical environments and observing how decisions are made in real time. This direct exposure helps developers grasp the contextual complexity of medical decision-making, from subtle diagnostic reasoning to emergency care pressures. When clinicians and developers work side by side, AI tools become smarter, more intuitive, and truly supportive of clinical expertise.
Training and AI Literacy for End Users
Even the most well-designed AI system will fail if clinicians do not adequately understand how to use it. Understanding a system’s limitations, challenging its results, and incorporating AI insights into human-driven decision-making are all components of AI literacy, which goes beyond simply knowing how to use a system.
To support this, developers should build in-tool training modules that guide users through interpreting AI recommendations. These modules should be easily accessible, integrated into the workflow, and tailored to different levels of AI familiarity.
Additionally, ongoing professional development in AI literacy should be encouraged. Hospitals and medical institutions can incorporate AI training into continuing education programs, ensuring clinicians remain critical thinkers in AI-assisted environments. Developers, in turn, should work with medical educators to create courses that teach how to evaluate AI-generated insights, recognize potential biases, and maintain accountability in decision-making.
Lessons Learned: Refining AI Through Iteration
The iterative refinement of AI models has proven crucial in preventing errors and enhancing usability. For example, a study on benzodiazepine de-prescribing revealed that clinicians occasionally misinterpreted AI-generated suggestions due to ambiguity in phrasing. When AI recommendations were unclear or lacked contextual explanation, clinicians defaulted to unnecessary caution or followed misleading guidance. This highlights a key takeaway for developers: clarity is as important as accuracy. Embedding contextual cues, confidence indicators, and alternative scenarios within AI outputs can significantly reduce misinterpretation.
Another breakthrough came from iterative design improvements in reflection-based AI systems. Early versions of an AI model provided direct recommendations, leading some users to accept them uncritically. When reflection prompts were introduced—questions that nudged clinicians to pause and verify AI-generated insights—the rate of blind acceptance dropped significantly. This simple yet powerful adjustment reinforced active engagement, proving that small interface changes can yield substantial improvements in clinical reasoning.
These examples highlight a recurring theme: AI works best when it facilitates rather than dictates medical decision-making. By learning from real-world deployments, developers can refine AI systems to foster critical thinking, transparency, and clinician autonomy, ensuring that AI remains a trusted partner in patient care efficiency.
Future Directions and Recommendations
The future of AI in healthcare depends not only on technological advancements, but also on how these tools evolve to better support clinical reasoning. As AI capabilities expand, developers must ensure that new innovations reinforce critical engagement, transparency, and human oversight. This requires both staying ahead of emerging trends and implementing concrete strategies for continuous improvement.
Emerging Trends in AI and Healthcare
One promising direction is using multi-agent AI systems, where multiple AI models interact to challenge each other’s outputs. This approach mimics clinical peer review, ensuring that AI-generated recommendations are cross-verified rather than presented as singular conclusions. By integrating these systems, developers can create AI that questions its own assumptions, reducing bias and encouraging clinicians to do the same.
Additionally, regulatory landscapes are shifting toward greater explainability and human-in-the-loop oversight. As AI becomes more integrated into clinical decision-making, developers must create systems that meet the growing demand for auditability and transparency. This includes ensuring that AI-generated insights remain interpretable, adjustable, and easy to scrutinize in high-stakes environments.
Actionable Recommendations for Developers
Developers should use a structured evaluation process to ensure that AI tools encourage critical thinking rather than passive acceptance. Below is a practical checklist for assessing AI system design:
✔ Does the AI present multiple recommendations rather than a single answer?
✔ Are explanations provided at different levels of detail based on user needs? (e.g., quick summaries for routine tasks, detailed rationales for complex cases)
✔ Can clinicians easily trace and verify AI outputs using source data?
✔ Does the interface encourage active reflection before accepting recommendations?
✔ Are built-in prompts, checklists, or confidence indicators reinforcing verification?
✔ How frequently are datasets audited for biases and inaccuracies?
✔ Is there a feedback mechanism for clinicians to refine AI recommendations?
Beyond initial design, continuous improvement is also essential. Developers should establish regular audits, user feedback loops, and iterative model updates to ensure AI remains clinically relevant and transparent. Training modules should be updated alongside system refinements, ensuring clinicians are equipped to use AI critically rather than dependently.
Final Thoughts
AI in healthcare should not be viewed as a static tool but as a collaborative system that evolves with real-world clinical input. The most effective AI models are refined through continuous clinician feedback, iterative testing, and ongoing validation. To keep clinicians engaged and accountable, developers must prioritize structured interactions such as step-by-step reasoning, confidence indicators, or built-in verification prompts.
The difficulty ultimately lies in finding a balance between efficiency and critical thinking. AI should streamline decision-making, but never at the cost of clinical judgment and responsibility.